目标跟踪之sort算法(3)
这里写目录标题
- 1 流程
- 1 预处理
- 2 跟踪
- 2 代码
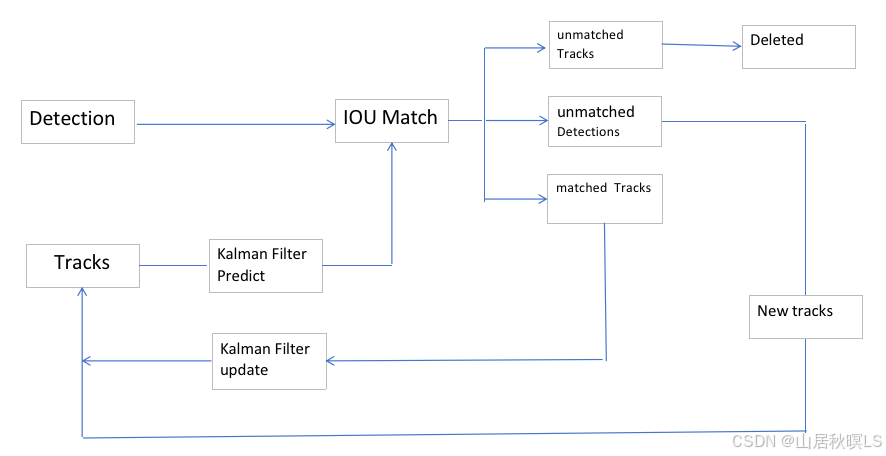
参考:sort代码 https://github.com/abewley/sort
1 流程
1 预处理
1.1 获取离线检测数据。1.2 实例化跟踪器。
2 跟踪
2.1 轨迹处理。根据上一帧的轨迹预测当前帧的轨迹,剔除到当前轨迹中为空的轨迹得到当前有效轨迹。
2.2 匹配。用匈牙利算法对有效轨迹和检测框匹配,得到匹配id、新检测id、未匹配ida. 如果跟踪器的个数为零,即第一帧图像,返回值为0的匹配id、新检测id、值为0的未匹配id。b. 如果跟踪器的个数为不为0,则计算检测框与当前轨迹的iou,如果iou不为空,得到iou大于阈值的掩码矩阵,判断掩码矩阵每行是否跟每列是一一对应,如果是则不需要匈牙利算法匹配;反之,用匈牙利算法得到匹配的检测框和轨迹的索引。c. 根据匹配索引得到新检测的框的id和为匹配的轨迹的id。d.根据iou再筛选一次。
2.3 更新轨迹。a. 对匹配上的轨迹,根据匹配id得到当前帧的最优估计。b. 添加新的检测。对于没有被匹配上的检测框生成新的跟踪器,并添加到轨迹中。c. 筛选轨迹。
2 代码
""" sort代码 https://github.com/abewley/sortSORT: A Simple, Online and Realtime TrackerCopyright (C) 2016-2020 Alex Bewley alex@bewley.aiThis program is free software: you can redistribute it and/or modifyit under the terms of the GNU General Public License as published bythe Free Software Foundation, either version 3 of the License, or(at your option) any later version.This program is distributed in the hope that it will be useful,but WITHOUT ANY WARRANTY; without even the implied warranty ofMERCHANTABILITY or FITNESS FOR A PARTICULAR PURPOSE. See theGNU General Public License for more details.You should have received a copy of the GNU General Public Licensealong with this program. If not, see <http://www.gnu.org/licenses/>.-i https://pypi.tuna.tsinghua.edu.cn/simple
filterpy==1.4.5
scikit-image==0.14.0
lap==0.4.0
numba==0.38.1
scikit-learn==0.19.1
"""
from __future__ import print_functionimport os
import numpy as np
import matplotlib
matplotlib.use('TkAgg')
import matplotlib.pyplot as plt
import matplotlib.patches as patches
from skimage import ioimport glob
import time
import argparse
from filterpy.kalman import KalmanFilternp.random.seed(0)def linear_assignment(cost_matrix):try:import lap_, x, y = lap.lapjv(cost_matrix, extend_cost=True)return np.array([[y[i],i] for i in x if i >= 0])except ImportError:from scipy.optimize import linear_sum_assignmentx, y = linear_sum_assignment(cost_matrix)return np.array(list(zip(x, y)))def iou_batch(bb_test, bb_gt):"""From SORT: Computes IOU between two bboxes in the form [x1,y1,x2,y2]"""bb_gt = np.expand_dims(bb_gt, 0)bb_test = np.expand_dims(bb_test, 1)xx1 = np.maximum(bb_test[..., 0], bb_gt[..., 0])yy1 = np.maximum(bb_test[..., 1], bb_gt[..., 1])xx2 = np.minimum(bb_test[..., 2], bb_gt[..., 2])yy2 = np.minimum(bb_test[..., 3], bb_gt[..., 3])w = np.maximum(0., xx2 - xx1)h = np.maximum(0., yy2 - yy1)wh = w * ho = wh / ((bb_test[..., 2] - bb_test[..., 0]) * (bb_test[..., 3] - bb_test[..., 1]) + (bb_gt[..., 2] - bb_gt[..., 0]) * (bb_gt[..., 3] - bb_gt[..., 1]) - wh) return(o) def convert_bbox_to_z(bbox):"""Takes a bounding box in the form [x1,y1,x2,y2] and returns z in the form[x,y,s,r] where x,y is the centre of the box and s is the scale/area and r isthe aspect ratio"""w = bbox[2] - bbox[0]h = bbox[3] - bbox[1]x = bbox[0] + w/2.y = bbox[1] + h/2.s = w * h #scale is just arear = w / float(h)return np.array([x, y, s, r]).reshape((4, 1))def convert_x_to_bbox(x,score=None):"""Takes a bounding box in the centre form [x,y,s,r] and returns it in the form[x1,y1,x2,y2] where x1,y1 is the top left and x2,y2 is the bottom right"""w = np.sqrt(x[2] * x[3])h = x[2] / wif(score==None):return np.array([x[0]-w/2.,x[1]-h/2.,x[0]+w/2.,x[1]+h/2.]).reshape((1,4))else:return np.array([x[0]-w/2.,x[1]-h/2.,x[0]+w/2.,x[1]+h/2.,score]).reshape((1,5))class KalmanBoxTracker(object):"""This class represents the internal state of individual tracked objects observed as bbox."""count = 0def __init__(self,bbox):"""Initialises a tracker using initial bounding box."""#define constant velocity modelself.kf = KalmanFilter(dim_x=7, dim_z=4) self.kf.F = np.array([[1,0,0,0,1,0,0],[0,1,0,0,0,1,0],[0,0,1,0,0,0,1],[0,0,0,1,0,0,0], [0,0,0,0,1,0,0],[0,0,0,0,0,1,0],[0,0,0,0,0,0,1]])self.kf.H = np.array([[1,0,0,0,0,0,0],[0,1,0,0,0,0,0],[0,0,1,0,0,0,0],[0,0,0,1,0,0,0]])self.kf.R[2:,2:] *= 10.self.kf.P[4:,4:] *= 1000. #give high uncertainty to the unobservable initial velocitiesself.kf.P *= 10.self.kf.Q[-1,-1] *= 0.01self.kf.Q[4:,4:] *= 0.01self.kf.x[:4] = convert_bbox_to_z(bbox)self.time_since_update = 0self.id = KalmanBoxTracker.countKalmanBoxTracker.count += 1self.history = []self.hits = 0self.hit_streak = 0self.age = 0def update(self,bbox):"""Updates the state vector with observed bbox."""self.time_since_update = 0self.history = []self.hits += 1self.hit_streak += 1 # 连续匹配并更新的次数self.kf.update(convert_bbox_to_z(bbox))def predict(self):"""Advances the state vector and returns the predicted bounding box estimate."""if((self.kf.x[6]+self.kf.x[2])<=0):self.kf.x[6] *= 0.0self.kf.predict()self.age += 1if(self.time_since_update>0): # 上一次更新距离现在的时间self.hit_streak = 0 # 匹配次数归0self.time_since_update += 1 # 轨迹只预测没有匹配的的次数➕1self.history.append(convert_x_to_bbox(self.kf.x))return self.history[-1]def get_state(self):"""Returns the current bounding box estimate."""return convert_x_to_bbox(self.kf.x)def associate_detections_to_trackers(detections,trackers,iou_threshold = 0.3):"""Assigns detections to tracked object (both represented as bounding boxes)Returns 3 lists of matches, unmatched_detections and unmatched_trackers"""if(len(trackers)==0):return np.empty((0,2),dtype=int), np.arange(len(detections)), np.empty((0,5),dtype=int)iou_matrix = iou_batch(detections, trackers)if min(iou_matrix.shape) > 0:a = (iou_matrix > iou_threshold).astype(np.int32)if a.sum(1).max() == 1 and a.sum(0).max() == 1:matched_indices = np.stack(np.where(a), axis=1) # 如果正好是一个检测与一个轨迹匹配,则找出匹配的索引else:matched_indices = linear_assignment(-iou_matrix) # 匈牙利匹配,matched_indices存储的是每个检测框对应的轨迹,第一列存储的是检测框的id;第二列存储的是检测框匹配的轨迹idelse:matched_indices = np.empty(shape=(0,2)) unmatched_detections = [] # 寻找没有被匹配上的检测框for d, det in enumerate(detections): # 这一步写的麻烦,不用枚举if(d not in matched_indices[:,0]):unmatched_detections.append(d)unmatched_trackers = [] # 寻找没有被匹配上的轨迹for t, trk in enumerate(trackers):if(t not in matched_indices[:,1]):unmatched_trackers.append(t)#filter out matched with low IOUmatches = [] # 寻找被匹配上的检测框的idfor m in matched_indices: # 根据iou再进行一次筛选if(iou_matrix[m[0], m[1]]<iou_threshold):unmatched_detections.append(m[0])unmatched_trackers.append(m[1])else:matches.append(m.reshape(1,2))if(len(matches)==0):matches = np.empty((0,2),dtype=int)else:matches = np.concatenate(matches,axis=0)return matches, np.array(unmatched_detections), np.array(unmatched_trackers)class Sort(object):def __init__(self, max_age=1, min_hits=3, iou_threshold=0.3):"""Sets key parameters for SORT"""self.max_age = max_ageself.min_hits = min_hitsself.iou_threshold = iou_thresholdself.trackers = []self.frame_count = 0def update(self, dets=np.empty((0, 5))):"""Params:dets - a numpy array of detections in the format [[x1,y1,x2,y2,score],[x1,y1,x2,y2,score],...]Requires: this method must be called once for each frame even with empty detections (use np.empty((0, 5)) for frames without detections).Returns the a similar array, where the last column is the object ID.NOTE: The number of objects returned may differ from the number of detections provided."""self.frame_count += 1# get predicted locations from existing trackers.trks = np.zeros((len(self.trackers), 5)) # 存储筛选后的轨迹。第一帧shape=(0, 5);to_del = [] # 没有匹配的轨迹ret = [] # 存放检测所有合格的轨迹for t, trk in enumerate(trks):pos = self.trackers[t].predict()[0] # 根据上一帧的轨迹当前帧的轨迹.trk[:] = [pos[0], pos[1], pos[2], pos[3], 0]if np.any(np.isnan(pos)):to_del.append(t)trks = np.ma.compress_rows(np.ma.masked_invalid(trks)) # 剔除当前无效轨迹for t in reversed(to_del):self.trackers.pop(t) # 剔除上一帧中的无效轨迹matched, unmatched_dets, unmatched_trks = associate_detections_to_trackers(dets,trks, self.iou_threshold) # 第一帧没有轨迹,# update matched trackers with assigned detections 对匹配的轨迹更新for m in matched: # 根据当前轨迹和检测得到当前最优估计self.trackers[m[1]].update(dets[m[0], :])# create and initialise new trackers for unmatched detectionsfor i in unmatched_dets: # 对于没有被匹配上的检测框生成新的跟踪器,并添加到轨迹中trk = KalmanBoxTracker(dets[i,:])self.trackers.append(trk)i = len(self.trackers)for trk in reversed(self.trackers):d = trk.get_state()[0]if (trk.time_since_update < 1) and (trk.hit_streak >= self.min_hits or self.frame_count <= self.min_hits): #(当前更新的轨迹)and (连续匹配超过min_hits or 检测帧数小于min_hits)ret.append(np.concatenate((d,[trk.id+1])).reshape(1,-1)) # +1 as MOT benchmark requires positivei -= 1# remove dead trackletif(trk.time_since_update > self.max_age):self.trackers.pop(i)if(len(ret)>0):return np.concatenate(ret)return np.empty((0,5))def parse_args():"""Parse input arguments."""parser = argparse.ArgumentParser(description='SORT demo')parser.add_argument('--display', dest='display', help='Display online tracker output (slow) [False]',action='store_true')parser.add_argument("--seq_path", help="Path to detections.", type=str, default='data')parser.add_argument("--phase", help="Subdirectory in seq_path.", type=str, default='train')parser.add_argument("--max_age", help="Maximum number of frames to keep alive a track without associated detections.", type=int, default=1)parser.add_argument("--min_hits", help="Minimum number of associated detections before track is initialised.", type=int, default=3)parser.add_argument("--iou_threshold", help="Minimum IOU for match.", type=float, default=0.3)args = parser.parse_args()return argsif __name__ == '__main__':# all trainargs = parse_args()display = args.display # 是否显示结果phase = args.phase # 'trian'total_time = 0.0 # 总时长total_frames = 0 # 记录检测的帧数colours = np.random.rand(32, 3) # \used only for display [32,3]if(display):if not os.path.exists('mot_benchmark'):print('\n\tERROR: mot_benchmark link not found!\n\n Create a symbolic link to the MOT benchmark\n (https://motchallenge.net/data/2D_MOT_2015/#download). E.g.:\n\n $ ln -s /path/to/MOT2015_challenge/2DMOT2015 mot_benchmark\n\n')exit()plt.ion()fig = plt.figure()ax1 = fig.add_subplot(111, aspect='equal')if not os.path.exists('output'):os.makedirs('output')pattern = os.path.join(args.seq_path, phase, '*', 'det', 'det.txt') # 相对路径 'data/train/*/det/det.txt'# 1. 数据准备for seq_dets_fn in glob.glob(pattern):mot_tracker = Sort(max_age=args.max_age, # 1.1 初始化跟踪器min_hits=args.min_hits,iou_threshold=args.iou_threshold) # create instance of the SORT trackerseq_dets = np.loadtxt(seq_dets_fn, delimiter=',') # 1.2 加载数据seq = seq_dets_fn[pattern.find('*'):].split(os.path.sep)[0] # 'data/train/ETH-Bahnhof/det/det.txt' --> ['ETH-Bahnhof', 'det', 'det.txt'] --> 'ETH-Bahnhof'with open(os.path.join('output', '%s.txt'%(seq)),'w') as out_file: # 'output/ETH-Bahnhof.txt'print("Processing %s."%(seq))for frame in range(int(seq_dets[:,0].max())): # seq_dets[:,0]第一列为图片的序列号,遍历每一帧的检测结果frame += 1 # detection and frame numbers begin at 1dets = seq_dets[seq_dets[:, 0]==frame, 2:7] # x1,y1,w,h,cdets[:, 2:4] += dets[:, 0:2] # convert to [x1,y1,w,h] to [x1,y1,x2,y2]total_frames += 1if(display):fn = os.path.join('mot_benchmark', phase, seq, 'img1', '%06d.jpg'%(frame))im = io.imread(fn)ax1.imshow(im)plt.title(seq + ' Tracked Targets')start_time = time.time()trackers = mot_tracker.update(dets) # 2. 获取跟踪结果cycle_time = time.time() - start_timetotal_time += cycle_timefor d in trackers: # 画的是跟踪到的轨迹print('%d,%d,%.2f,%.2f,%.2f,%.2f,1,-1,-1,-1'%(frame,d[4],d[0],d[1],d[2]-d[0],d[3]-d[1]),file=out_file)if(display):d = d.astype(np.int32)ax1.add_patch(patches.Rectangle((d[0],d[1]),d[2]-d[0],d[3]-d[1],fill=False,lw=3,ec=colours[d[4]%32,:]))if(display):fig.canvas.flush_events()plt.draw()ax1.cla()print("Total Tracking took: %.3f seconds for %d frames or %.1f FPS" % (total_time, total_frames, total_frames / total_time))if(display):print("Note: to get real runtime results run without the option: --display")
相关文章:
目标跟踪之sort算法(3)
这里写目录标题 1 流程1 预处理2 跟踪 2 代码 参考:sort代码 https://github.com/abewley/sort 1 流程 1 预处理 1.1 获取离线检测数据。1.2 实例化跟踪器。2 跟踪 2.1 轨迹处理。根据上一帧的轨迹预测当前帧的轨迹,剔除到当前轨迹中为空的轨迹得到当前…...
C++/stack_queue
目录 1.stack 1.1stack的介绍 1.2stack的使用 练习题: 1.3stack的模拟实现 2.queue的介绍和使用 2.1queue的介绍 2.2queue的使用 2.3queue的模拟实现 3.priority_queue的介绍和使用 3.1priority_queue的介绍 3.2priority_queue的使用 欢迎 1.stack 1.1stack…...
小程序电商运营内容真实性增强策略及开源链动2+1模式AI智能名片S2B2C商城系统源码的应用探索
摘要:随着互联网技术的不断发展,小程序电商已成为现代商业的重要组成部分。然而,如何在竞争激烈的市场中增强小程序内容的真实性,提高用户信任度,成为电商运营者面临的一大挑战。本文首先探讨了通过图片、视频等方式增…...
「Unity3D」在Unity中使用C#控制显示Android的状态栏
Unity打包的Android默认都是全屏,如果想要在真机上显示状态栏,就需要额外设置,有两种方式: 第一种,使用Android的Java代码去控制,然后以插件的方式放到Unity中,被C#调用。第二种,使…...
基于51单片机和ESP8266(01S)、LCD1602、DS1302、独立按键的WiFi时钟
目录 系列文章目录前言一、效果展示二、原理分析三、各模块代码1、延时2、定时器03、串口通信4、DS13025、LCD16026、独立按键 四、主函数总结 系列文章目录 前言 之前做了一个WiFi定时器时钟,用八位数码管进行显示,但是定时器时钟的精度较低࿰…...
AI 浪潮席卷中国年,开启科技新春新纪元
在这博主提前祝大家蛇年快乐呀!!! 随着人工智能(AI)技术的飞速发展,其影响力已经渗透到社会生活的方方面面。在中国传统节日 —— 春节期间,AI 技术也展现出了巨大的潜力,为中国年带…...
STM32 LED呼吸灯
接线图: 这里将正极接到PA0引脚上,负极接到GND,这样就高电平点亮LED,低电平熄灭。 占空比越大,LED越亮,占空比越小,LED越暗 PWM初始化配置 输出比较函数介绍: 用这四个函数配置输…...
机器学习day4
自定义数据集 使用pytorch框架实现逻辑回归并保存模型,然后保存模型后再加载模型进行预测 import numpy as np import torch import torch.nn as nn import torch.optim as optimizer import matplotlib.pyplot as pltclass1_points np.array([[2.1, 1.8],[1.9, 2…...
《哈佛家训》
《哈佛家训》是一本以教育为主题的书籍,旨在通过一系列富有哲理的故事和案例,传递积极的人生观、价值观和教育理念。虽然它并非直接由哈佛大学官方出版,但其内容深受读者喜爱,尤其是在家庭教育和个人成长领域。 以下是《哈佛家训…...
为什么redis会开小差?Redis 频繁异常的深度剖析与解决方案
文章目录 导读为什么redis会开小差?1.连接数过多2.bigkey3.慢命令操作4.内存策略不合理5.外部数据双写一致性6.保护机制未开启7. 数据集中过期8. CPU饱和9. 持久化阻塞10. 网络问题结论 导读 提起分布式缓存,想必大多数同学脑海中都会浮出redis这个名字…...
window中80端口被占用问题
1,查看报错信息 可以看到在启动项目的时候,8081端口被占用了,导致项目无法启动。 2,查看被占用端口的pid #语法 netstat -aon|findstr :被占用端口#示例 netstat -aon|findstr :8080 3,杀死进程 #语法 taikkill /pid…...
< OS 有关 > 阿里云:轻量应用服务器 的使用 :轻量化 阿里云 vpm 主机
原因: < OS 有关 > 阿里云:轻量应用服务器 的使用 :从新开始 配置 SSH 主机名 DNS Tailscale 更新OS安装包 最主要是 清除阿里云客户端这个性能杀手-CSDN博客 防止 I/O 祸害系统 操作: 查看进程&#x…...
解决报错“The layer xxx has never been called and thus has no defined input shape”
解决报错“The layer xxx has never been called and thus has no defined input shape”(这里写自定义目录标题) 报错显示 最近在跑yolo的代码时遇到这样一个错误,显示“the layer {self.name} has never been called”.这个程序闲置了很久,每次一遇到…...
Microsoft Visual Studio 2022 主题修改(补充)
Microsoft Visual Studio 2022 透明背景修改这方面已经有很多佬介绍过了,今天闲来无事就补充几点细节。 具体的修改可以参考:Microsoft Visual Studio 2022 透明背景修改(快捷方法)_material studio怎么把背景弄成透明-CSDN博客文…...
PyCharm接入DeepSeek实现AI编程
目录 效果演示 创建API key 在PyCharm中下载CodeGPT插件 配置Continue DeepSeek 是一家专注于人工智能技术研发的公司,致力于开发高性能、低成本的 AI 模型。DeepSeek-V3 是 DeepSeek 公司推出的最新一代 AI 模型。其前身是 DeepSeek-V2.5,经过持续的…...
C++ 包装器与绑定器的应用之回调函数的实现
回调函数的实现 在消息队列和网络库的框架中,当接收到消息(报文)时,回调用户自定义的函数对象,把消息(报文)参数传给它,由它决定如何处理。 queue参考文章:C queue(STL queue&…...
一文读懂DeepSeek-R1论文
目录 论文总结 摘要 1. 引言 1.1. 贡献 1.2. 评估结果总结 2. 方法 2.1. 概述 2.2. DeepSeek-R1-Zero:在基础模型上进行强化学习 2.2.1. 强化学习算法 2.2.2. 奖励建模 2.2.3. 训练模板 2.2.4. DeepSeek-R1-Zero 的性能、自我进化过程和顿悟时刻 2.3. …...
文献阅读 250128-Tropical forests are approaching critical temperature thresholds
Tropical forests are approaching critical temperature thresholds 来自 <Tropical forests are approaching critical temperature thresholds | Nature> 热带森林正在接近临界温度阈值 ## Abstract: The critical temperature beyond which photosynthetic machinery…...
【python】python基于机器学习与数据分析的二手手机特性关联与分类预测(源码+数据集)【独一无二】
👉博__主👈:米码收割机 👉技__能👈:C/Python语言 👉专__注👈:专注主流机器人、人工智能等相关领域的开发、测试技术。 python基于机器学习与数据分析的二手手机特性关联与…...
构建可靠的时间序列预测模型:数据泄露检测、前瞻性偏差消除与因果关系验证
在时间序列分析领域中,存在多种可能影响分析结果有效性的技术挑战。其中,数据泄露、前瞻性偏差和因果关系违反是最为常见且具有显著影响的问题。 数据泄露:隐蔽的系统性错误 数据泄露是指在预测时理论上无法获取的信息,通过某种…...
Spring Boot - 数据库集成05 - 集成MongoDB
Spring Boot集成MongoDB 文章目录 Spring Boot集成MongoDB一:使用前的准备1:依赖导入 & 配置2:实体类创建 二:核心 - MongoRepository三:核心 - MongoTemplate1:集合操作2:文档操作(重点)3&…...
计算机网络之运输层
本文章目录结构出自于《王道计算机考研 计算机网络_哔哩哔哩_bilibili》 04 传输层 在网上看到其他人做了相关笔记,就不再多余写了,直接参考着学习吧。 王道考研 计算机网络笔记 第五章:传输层_王道计算机网络传输层_Baret-H的博客-CSDN博…...
DeepSeekMoE:迈向混合专家语言模型的终极专业化
一、结论写在前面 论文提出了MoE语言模型的DeepSeekMoE架构,目的是实现终极的专家专业化(expert specialization)。通过细粒度的专家分割和共享专家隔离,DeepSeekMoE相比主流的MoE架构实现了显著更高的专家专业化和性能。从较小的2B参数规模开始&#x…...
知识库管理驱动企业知识流动与工作协同创新模式
内容概要 知识库管理在现代企业中扮演着至关重要的角色,其价值不仅体现在知识的积累,还在于通过优质的信息流动促进协作与创新。有效的知识库能够将分散的信息整合为有序、易于访问的资源,为员工提供实时支持,进而提升整体工作效…...
二叉树-堆(补充)
二叉树-堆 1.二叉树的基本特性2.堆2.1.堆的基本概念2.2.堆的实现2.2.1.基本结构2.2.2.堆的初始化2.2.3.堆的销毁2.2.4.堆的插入2.2.5.取出堆顶的数据2.2.6.堆的删除2.2.7.堆的判空2.2.8.堆的数据个数2.2.9.交换2.2.10.打印堆数据2.2.11.堆的创建2.2.12.堆排序2.2.13.完整代码 3…...
Java面试题2025-并发编程基础(多线程、锁、阻塞队列)
并发编程 一、线程的基础概念 一、基础概念 1.1 进程与线程A 什么是进程? 进程是指运行中的程序。 比如我们使用钉钉,浏览器,需要启动这个程序,操作系统会给这个程序分配一定的资源(占用内存资源)。 …...
【方法论】ChatGPT与DeepSeek的联合应用,提升工作效率的新解决方案
标题:ChatGPT与DeepSeek的联合应用,提升工作效率的新解决方案 【表格】ChatGPT与DeepSeek联合应用流程 阶段工具主要任务优势备注初稿生成ChatGPT基于用户输入生成初步内容高效、快速生成内容,适应多种主题适合生成长篇文章、报告、分析等验…...
RoboMaster- RDK X5能量机关实现案例(一)识别
作者:SkyXZ CSDN:https://blog.csdn.net/xiongqi123123 博客园:https://www.cnblogs.com/SkyXZ 在RoboMaster的25赛季,我主要负责了能量机关的视觉方案开发,目前整体算法已经搭建完成,实际方案上我使用的上…...
5分钟带你获取deepseek api并搭建简易问答应用
目录 1、获取api 2、获取base_url和chat_model 3、配置模型参数 方法一:终端中临时将加入 方法二:创建.env文件 4、 配置client 5、利用deepseek大模型实现简易问答 deepseek-v3是截止博文撰写之日,无论是国内还是国际上发布的大模型中…...
Ikigai是什么
Ikigai(生き甲斐) 是一个日语词语,意思是“生活的意义”或“生命的价值所在”。它是一种关于人生意义的哲学概念,源自日本文化,强调通过找到自己热爱、擅长、社会需要以及能带来经济回报的交集来实现幸福和满足感。 I…...
基于PyQt设计的智能停车管理系统
文章目录 一、前言1.1 项目介绍【1】项目开发背景【2】设计实现的功能【3】设计意义【4】国内外研究现状【6】摘要1.2 设计思路1.3 系统功能总结1.4 开发工具的选择【1】VSCODE【2】python【3】ptqt【4】HyperLPR31.5 参考文献二、安装Python环境1.1 环境介绍**1.2 Python版本介…...
Flink (十二) :Table API SQL (一) 概览
Apache Flink 有两种关系型 API 来做流批统一处理:Table API 和 SQL。Table API 是用于 Scala 和 Java 语言的查询API,它可以用一种非常直观的方式来组合使用选取、过滤、join 等关系型算子。Flink SQL 是基于 Apache Calcite 来实现的标准 SQL。无论输入…...
MySQL知识点总结(十三)
执行逻辑备份要具备哪些条件,其优缺点在哪。 逻辑备份是温备,创建逻辑备份文件时,MySQL服务器必须处于运行状态,其他应用程序在逻辑备份期间不能修改但可以执行读取操作。逻辑备份会把表结构和数据转换为SQL语句保存。 逻辑备份…...
ACL-2024 | 具身智能空间理解能力几何?EmbSpatial-Bench:视觉语言大模型在具身任务中空间理解水平测试基准
作者:Mengfei Du, Binhao Wu, Zejun Li, Xuanjing Huang, Zhongyu Wei 单位:复旦大学数据科学学院,复旦大学计算机科学学院 论文标题:EmbSpatial-Bench: Benchmarking Spatial Understanding for Embodied Tasks with Large Vis…...
动手学图神经网络(6):利用图神经网络进行点云分类
利用图神经网络进行点云分类 引言 在本教程中,大家将学习使用图神经网络(Graph Neural Networks, GNN)进行点云分类的基本工具。给定一组对象或点集的数据集,将这些对象嵌入到一个特征空间中,使得它们在特定任务下能够分类。将原始点云作为神经网络的输入,让网络学习捕…...
Ollama+DeepSeek本地大模型部署
1、Ollama 官网:https://ollama.com/ Ollama可以干什么? 可以快速在本地部署和管理各种大语言模型,操作命令和dokcer类似。 mac安装ollama: # 安装ollama brew install ollama# 启动ollama服务(默认11434端口…...
docker安装Redis:docker离线安装Redis、docker在线安装Redis、Redis镜像下载、Redis配置、Redis命令
一、镜像下载 1、在线下载 在一台能连外网的linux上执行docker镜像拉取命令 docker pull redis:7.4.0 2、离线包下载 两种方式: 方式一: -)在一台能连外网的linux上安装docker执行第一步的命令下载镜像 -)导出 # 导出镜像…...
HTML 标题
HTML 标题 引言 HTML(超文本标记语言)是构建网页的基础,而标题则是网页中不可或缺的元素。标题不仅能够帮助用户快速了解网页内容,还能够对搜索引擎优化(SEO)产生重要影响。本文将详细介绍HTML标题的用法…...
记录 | MaxKB创建本地AI智能问答系统
目录 前言一、重建MaxKBStep1 复制路径Step2 删除MaxKBStep3 创建数据存储文件夹Step4 重建 二、创建知识库Step1 新建知识库Step2 下载测试所用的txtStep3 上传本地文档Step4 选择模型补充智谱的API Key如何获取 Step5 查看是否成功 三、创建应用Step1 新建应用Step2 配置AI助…...
Linux 非阻塞IO
Linux 非阻塞IO 1. fcntl() 在Linux操作系统中,fcntl() 是一个用于操作文件描述符的系统调用。它提供了多种功能,包括控制文件描述符的属性、管理文件锁定、设置文件的非阻塞模式等。 本文只截取了用于IO模型的 fcntl() 部分内容, fcntl() …...
美国本科申请文书PS写作中的注意事项
在完成了introduction之后,便可进入到main body的写作之中。美国本科申请文书PS的写作不同于学术论文写作,要求你提出论点进行论证之类。PS更多的注重对你自己的经历或者motivation的介绍和描述。而这一描述过程只能通过对你自己的过往的经历的展现才能体…...
Qt文件操作
目录 一、文件操作相关类 1.QFile 2.QFileInfo 3.QTextStream 4.QDataStream 5.QDir 6.QFileSystemWatcher 7.QTemporaryFile 二、文件操作示例 1.文本文件操作 2.目录操作 3.二进制文件操作 一、文件操作相关类 1.QFile QFile类用于文件的创建、读写、复制、删除…...
赚钱的究极认识
1、赚钱的本质是提供了价值或者价值想象 价值: 比如小米手机靠什么?“性价比”,什么饥饿营销,创新,用户参与,生态供应链,品牌这些不能说不重要,但是加在一起都没有“性价比”这3字重…...
【项目】基于Qt开发的音乐播放软件
目录 项目介绍 项目概述 界面开发 界面分析 创建工程 主界面布局设计 窗口主框架设计 界面美化 主窗口设定 添加图片资源 head处理 播放控制区处理 自定义控件 BtForm 推荐页面 自定义CommonPage 自定义ListItemBox 自定义MusicSlider 自定义VolumeTool 音…...
week08_文本匹配任务
1、文本匹配任务概述 狭义: 给定一组文本,判断其是否语义相似 今天天气不错 match 今儿个天不错呀 √ 今天天气不错 match 你的代码有bug 以分值形式给出相似度 今天天气不错 match 今儿个天不错呀 0.9 今天天气不错 match…...
01-01 五元组
[外链图片转存中…(img-8JR8fhPZ-1737855365022)] 01-01 五元组 网络中的五元组(5-Tuple) 是用于唯一标识一个网络连接或数据流的五个关键参数组合。这五个参数共同定义了数据包的来源、目的地以及传输方式,是网络设备(如防火墙…...
5.2 软件需求分析
文章目录 需求分析的意义软件需求的组成需求分析的5个方面需求分析方法 需求分析的意义 需求分析解决软件“做什么”的问题。由于开发人员比较熟悉计算机而不熟悉领域业务,用户比较熟悉领域业务而不熟悉计算机,双方需要通过交流,制定出完整、…...
OpenCV:在图像中添加噪声(瑞利、伽马、脉冲、泊松)
目录 简述 1. 瑞利噪声 2. 伽马噪声 3. 脉冲噪声 4. 泊松噪声 总结 相关阅读 OpenCV:在图像中添加高斯噪声、胡椒噪声-CSDN博客 OpenCV:高通滤波之索贝尔、沙尔和拉普拉斯-CSDN博客 OpenCV:图像处理中的低通滤波-CSDN博客 OpenCV&…...
进程池的制作(linux进程间通信,匿名管道... ...)
目录 一、进程间通信的理解 1.为什么进程间要通信 2.如何进行通信 二、匿名管道 1.管道的理解 2.匿名管道的使用 3.管道的五种特性 4.管道的四种通信情况 5.管道缓冲区容量 三、进程池 1.进程池的理解 2.进程池的制作 四、源码 1.ProcessPool.hpp 2.Task.hpp 3…...
C++:多继承习题3
题目内容: 声明一个时间类Time,时间类中有3个私有数据成员(Hour,Minute,Second)和两个公有成员函数(SetTime和PrintTime)。要求: (1) SetTime根据传递的3个参数为对象设置时间; &a…...